The index to the articles in this series is found here.
OK, I’m not going to turn this series of posts into a stack of plots of loss vs. epoch number, that’s not why we’re here. There is, however, one more I’d like to show. In the last post we saw some training results that were strongly suggestive of overfitting. I’m going to show one here that is unambiguous. This is the Adagrad optimizer in Keras, with default settings and batch size. This optimizer is a good choice for fitting sparse data. Even after thinning our dataset to remove many uninteresting inputs, any particular bit in the output set is still fairly sparse, so this seems a promising candidate.
Plotting the losses against epoch number again, we see this:
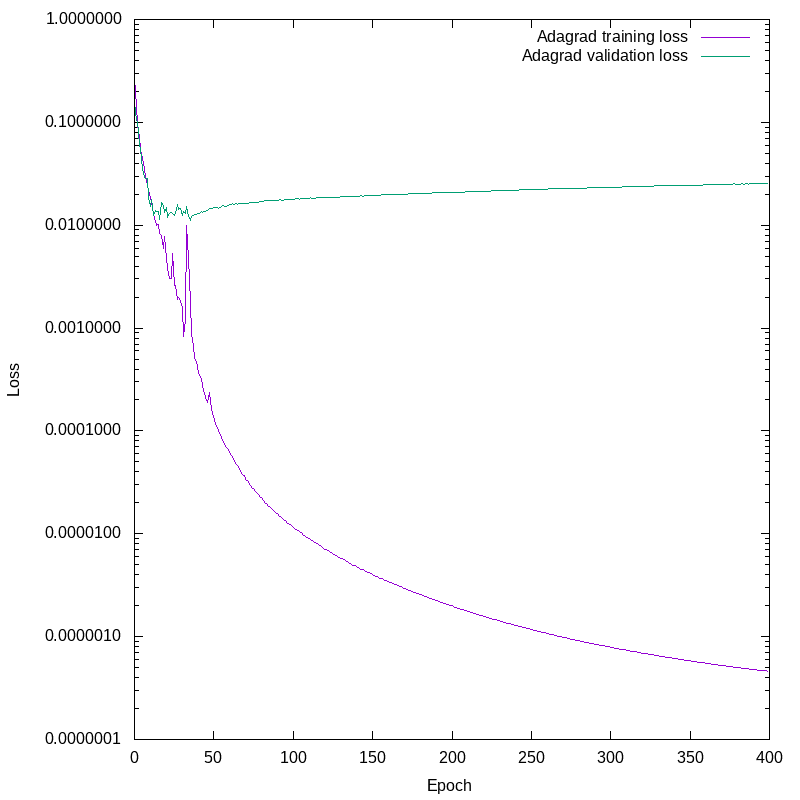
This is unambiguous. Note the logarithmic scale on the Y axis. Fairly early on, the validation loss detaches from the training loss. The network continues to improve the training loss by four decades, while the validation loss slowly increases. From about epoch 20 onward we are not improving the network’s ability to predict the future given new data, we are only getting it more and more obsessively correct about the training set, and our predictions on novel data are worsening.
All right, I’ve got four more optmizers bundled with Keras to test. I won’t post about those in detail unless something new appears, interesting in a different way. Once I’ve run through all the optimizers, I’ll present some other data about how well the resulting networks performed.